A Calm Discussion about GF% and xGF%
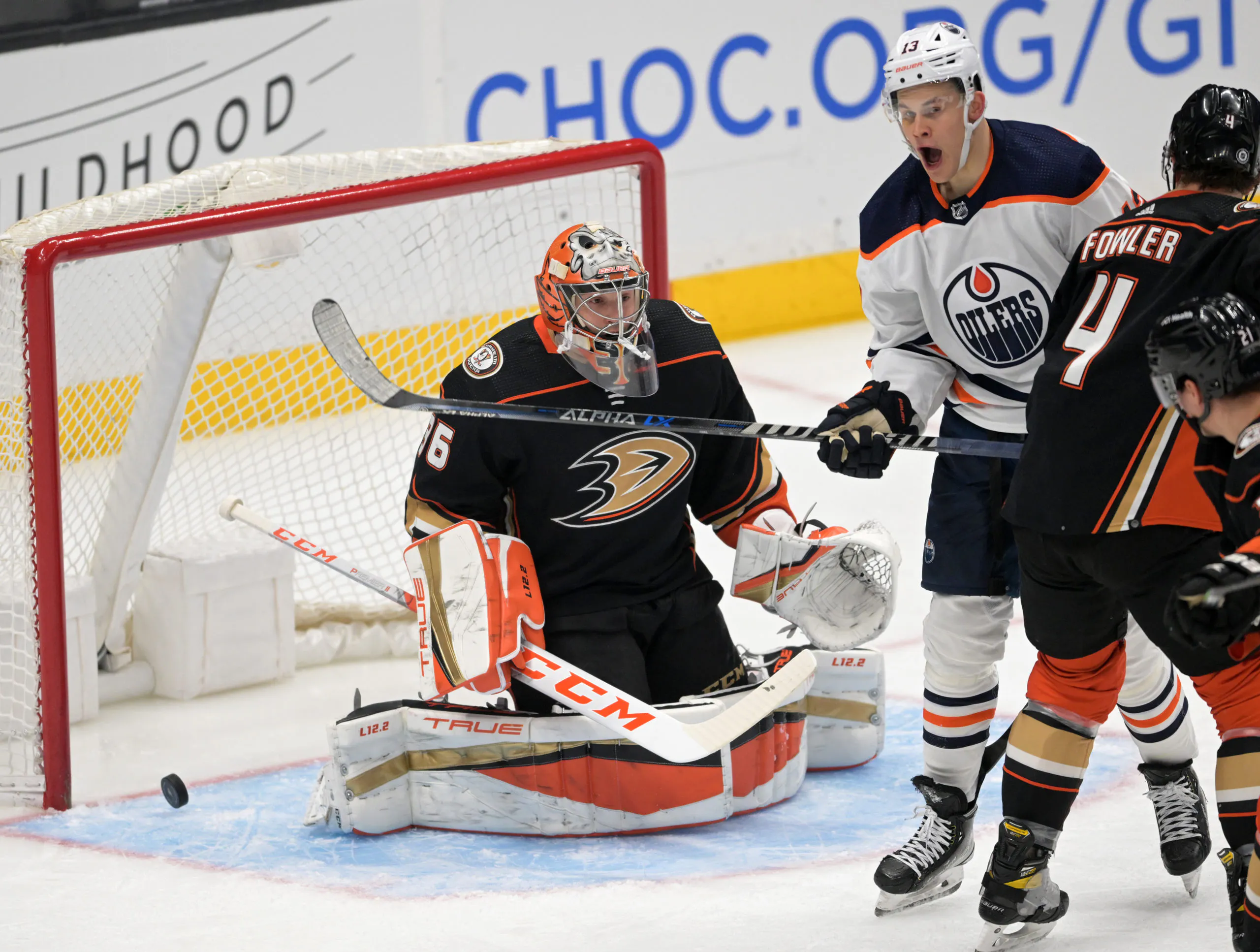
By Jason Gregor
2 years agoThere will always be extreme view points on any topic, but they often aren’t how the majority feel. Whether that is politics, education, health or sports, the extreme ends of the spectrum are usually the vocal minority. That is no different in NHL discussions when evaluating and analyzing players. If everyone always agreed it would be boring, and I’d never expect all fans or analysts to see players in the same light. The adage “One person’s garbage is another person’s treasure” is keenly accurate when discussing players.
A few weeks ago I outlined the most polarizing player in Edmonton right now, Jesse Puljujarvi. I believe the vast majority of people see the strengths and weaknesses of his game, and will favour one side. Then you have the extreme ends of the spectrum. One end claims he is terrible, while the other end pumps up his analytics to suggest he’s elite. He is neither, and I sense the majority recognize this and their analyses of him sit slightly left or right of centre (a contributing NHL player).
I won’t rehash the article here. You can read and come to your own conclusion, but the Puljujarvi debate has raged on for years, often around other players. Instead of discussing those players I wanted to discuss GF%, xGF% and more with a few people whose analyses on players I enjoy. We don’t always agree, which is fine, as every fan or analyst will have their own personal biases. Once we acknowledge that it makes for a better discussion/debate.
I reached out to Mike Kelly from Sport Logiq and the NHL Network, Woodguy from PuckIQ.com and Sid from Oilersnation and they answered four questions.
Question: What do you value more? GF% or xGF% or both, and why?
@MikeKellyNHL: Both are important. I place more value on expected goal differential in terms of understanding how well a player, a line or a team is playing from a process standpoint. This tends to affect GF% more than vice-versa. Over long samples, GF% will paint a fairly accurate picture but small sample, xGF% more indicative of play. It also eliminates the massive variable that is goaltending at both ends of the ice.
@NHL_Sid: Personally, I like to split up GF% into GF and GA, xGF% into xGF and xGA, and go from there. In single-season samples, I use GF, xGF and xGA for forwards, and primarily value xGF and xGA for defencemen.
I did some research a while back, but barring certain elite offensive defencemen, most D-men tend to have low year-to-year repeatability in regards to GF. Defencemen have a much smaller impact on on-ice shooting ability than forwards, which is why I use xGF for both, but place more emphasis on GF for forwards. However, over a multi-season sample, GF can be used for defencemen; it’s difficult to say a defenceman has been “unlucky” for significant TOI in several seasons. As for my thoughts on GA, I like to use this analogy:
Let’s say we have Player A, who’s unanimously considered elite defensively. For one half of the season, place Player A on a team with a Vezina-calibre goalie. For the other half, place him on a team with a fringe ECHL goalie. Let’s say Player A sustains an elite xGA impact for the entire season, his GA is also at an elite level in the first half of the season, but his GA considerably declines in the second half. Is this decline in GA Player A’s fault, even though his chance suppression rate consistently remained excellent? Or is it due to the change in goaltending?
In this example, it’s pretty obvious it’s the latter IMO. My definition of skater defence is to make your goalie’s workload as easy as possible, by preventing as many chances against as possible, but it isn’t entirely your fault if the goalie fails to make the saves in the first place. Unless a player’s GA is close to their xGA, it can be unfair to place a lot of emphasis on GA, as skaters have minimal control on goaltending performance. Their individual defensive capabilities shouldn’t be affected by the capabilities of their goaltending. With that said, if a player consistently overachieves or underachieves their GA over several seasons, this trend may be a sign that public xGA is somehow overrating or underrating them.
Overall, I value xGF% more in smaller/single-season samples due to the fact that luck is very common in hockey, and xGF% can give a better idea of a player’s sustainable impact/capabilities. However, GF% should certainly be used over a significant multi-season sample.
@Woodguy55: I value both for different reasons as they measure different things. I value GF% in large samples, like 60+ games, but multiple seasons is better. Winning the goal share is everything as it determines who wins the game.
GF% is strongly influenced by shooting percentage and save percentage which can fluctuate wildly in small samples so it’s best to look at big samples. Forwards also have next to no impact on SV% so that is out of their control. Any player who can help drive positive goal share with his teammates is very valuable as it’s not a common trait in the NHL as everyone is very good.
It is also best to see how a player impacts his team mates’ on ice GF%. Does he help it or hurt it? Good players on teams with bad goaltending and iffy D-corps can have lower GF% due to problems beyond their control but they will still impact their teammates by improving their GF% when they are on the ice with him.
GF% can have more meaning in larger samples as the ability to put the puck in the net, and create opportunities for goal scoring is a real skill that doesn’t get measured well by xGF%. I value xGF% because it’s a “flow of play” result. It measures shot volume weighted by shot location and shot type. This measures which team had the puck in the ozone more and took more shots. The best way to not get scored on is to be in the ozone as much as possible.
We’ve all watched games and thought “my team deserved to win” when they owned the puck all game, but lost. This is what xGF% measures. “Who owns the puck”? Very good teams come out with a xGF% of ~54% or so. Like GF%, a single xGF% number doesn’t mean much. What is much more meaningful is the impact a player has on his teammate’s xGF%. In smaller samples you can see which players “tilt the ice” and help their team create more offensive opportunities.
Smart teams like FLA seem to pluck players like Reinhart and Duclair off of teams (or sign as FAs) who have good to very good impact on their team mates GF% and xGF%. There is value in examining these results.
Question: If we added GF% and xGF% together would those two totals be a good combination to illustrate what a player is doing at 5×5?
@MikeKellyNHL: I’ve actually done this, not as a final analysis, but as a starting point when looking at how effective a line has been. A great process is one thing but at the end of the day, players still need to be able to put the puck in the net. There are players who over and underperform their expected goal totals, year-over-year for different reasons. Auston Matthews will always score more than his expected goal total because he is an elite scorer who can convert on chances most others can’t. Brady Tkachuk is an example of someone who has historically underperformed. He gets a lot of high expected goal value shots as he’s one of the best producing shots from in tight but if you watch, a lot end up getting jammed into a goalies pads. Combining the two leads to an obvious multicollinearity issue but again, not a final evaluation method — a more granular way to get to the next step.
@NHL_Sid: In regards to simply adding GF% and xGF% together, I’m not sure how efficient of a method that would be. As I explained in my previous answer, I don’t think GF, GA, xGF, xGA should all have equal weightage and emphasis, especially as offence typically matters more than defence (although both are obviously important).
Additionally, I think adding some sort of teammates, competition and shift start adjustment would also be important, as opposed to adding raw GF% and xGF% together. Deployment context is crucial.
@Woodguy55: I don’t see there being any value in that. They measure different things. It’s good to look at both for sure, but I don’t see value in combining them. xGF% and GF% analysis is best done by seeing how a player impacts their team mates and combining both types of results would make that near impossible.
Question: Do you have a formula, or have you seen one, that accurately reflects value of GF% to xGF%? Is one GF equal to one xGF?
@MikeKellyNHL: No and it would defeat the purpose in a way. Or, at least be a different approach. It’s difficult to produce a shot with an expected goal value north of about 70 percent. Breakaways and seam-passes, one-timers from the low slot are two of the highest probability chances a player can produce and those still don’t go in most of the time. The benefit of using xGF% as an evaluation tool isn’t to put all of your stock in it. It is to illuminate. If a line or player shows well or poorly, it’s the first chapter into understanding why that is. It is not the final chapter to determine if that player is ‘good’ or ‘bad.’ Think of how many players succeed in certain environments and fail in others. Goaltending is a massive variable in GF% which is why using xGF% is a necessary evaluation tool.
@NHL_Sid: Interesting question. I don’t think you can make a fixed statement such as “One goal = __ shot attempts” since simple shot attempts vary in quality. For example, taking 10 low-quality point-shots shouldn’t be considered equal to taking 10 high-danger shots off the rush or in the slot.
The main point of xG models is to predict exact goal values as accurately as possible, so one GF is essentially supposed to equal one xGF, especially since xGF attempts to take into account both quality and quantity. I think something like “One goal = __ high danger chances” would be even more interesting. Per NST, there’s been roughly ~4.34 high danger chances per goal scored this season. Would certainly be something to look into in more detail (although I’m not certain as to how accurate NST’s HDCF model is).
@Woodguy55: There is no real formula because different players will have long term results. Some players drive high SH% so their actual GF% will usually be higher than their xGF% over bigger samples. Other players will play in front of terrible goaltending (see NJD this year) and their xGF% will be higher than their GF%. Conversely when Lundqvist played for NYR their GF% usually beat the xGF% handily as the usually had the best SV% in the league.
There are many variables to consider so “easy formulas” or “single output results” from models are not a good way to use this information to evaluate players. Context is everything and there is a lot to consider.
Question: There is a lot of focus on 5×5 play, but considering goals have the largest impact in the outcome in games, and many are scored on special teams, should we focus more on PP and PK contributions from players?
@MikeKellyNHL: That’s a subjective question. I certainly focus a lot on special teams, so in that sense, I agree with you. 5×5 play is the most normalized game state where all players play and a majority of the game is played so there are obvious benefits to evaluating players in this game state across the board. However, special teams are a critical component of the game. If two players are comparable 5×5 and both play power play or kill penalties and one is better than the other at it, that’s useful information.
@NHL_Sid: 5v5 play matters the most by a considerable margin, but I think PP value can be exceedingly undervalued at times, such as instances where certain fans discredit PP production for players like McDavid and Draisaitl. I think excelling on the PP is a genuinely valuable and important skill.
To a lesser degree, penalty killing can also be important, but it matters much less than 5v5 Offence, 5v5 Defence, and PP Offence. Not to mention, power plays are heavily reliant on puck movement as opposed to 5v5, and public xG models don’t have access to that kind of data. Consequently, I don’t think there’s many good publicly available tools to accurately assess penalty killing in the first place, besides GA in bigger samples. This is also why I currently use GF and points to assess PP play, and rarely place emphasis on PP xGF, at least until we have access to private/pre-shot movement data.
@Woodguy55: I understand why there is a focus on 5v5 play because 83% of the game is played at Even Strength (5v5, 4v4, 3v3), and about 70% of goals scored in a game come at even strength (not including empty net goals).
Five-on-five is also the toughest situation to drive goal share and shot share, so it is highly valued by many people. If a player can dominate 5v5 that’s critical to a team’s success. It is the vast majority of the TOI and a big majority of the goals scored in a game. That being said, hockey games are decided by goals and driving goal share/goal differential in all game states, including on the PP, is important.
Power play TOI account for about 8% of total TOI in the average hockey game, but PP goals account for about 19% of the total goals (not including shorties) scored in a hockey game. Ignoring or minimizing roughly 20% of the goals scored in the NHL isn’t good analysis. You need to include power play goal share/differential as well as EV and weight them accordingly.
WRAP UP…
We will never all see a player the same. There is lots of data available, and if you like or dislike a player you will be able to find one stat to support that viewpoint. It is easy to find one, but can you find multiple? That is the challenge and compiling the most information, whether it be stats, analytics or video to support our viewpoint often is best.
Mike added this statement that I think is very accurate and a good reminder:
“An important lesson I’ve learned is to not overvalue any one metric or model,” said Kelly. “There is no perfect way to evaluate a player. This goes for traditional scouting methods and by quantifying as much as possible of what a player does on the ice. There is value, over a large enough sample in looking at a players GF% and there is value in looking at a player’s xGF%.
“Independently, and combined, neither as a final evaluation of who that player is or how they perform. I often liken it to peeling an onion. A player has a good GF%, why is that? Let’s look at the process underneath the results — xGF% — also strong, why is that? Let’s look at the individual contributions — offensively, defensively, transitioning the puck, managing the puck, winning the puck back etc. Will a player succeed in one system despite failing in another? There are examples of this every year. Calgary’s third defence pair of Nikita Zadorov and Erik Gudbranson are a good example. I am a lot more interested in best understanding who a player is. From there you can determine how they can best be successful.”
How a coach deploys a player, with who, and in what system can impact their success. The best scouts and GMs will sign players whose skillsets will match with how they want to play. Every off-season we see teams sign players to contracts that are destined to fail. Don’t ask players to be what they’re not. Know their strengths and deploy them in situations which are best suited for them to have success.
Heading into free agency and the draft week, where trades are more prevalent, it will be interesting to see who signs, or acquires players, best suited to fill a role on their new team, and how many teams sign or acquire players, who they hope can fill the spot. To me there is a big difference, and the teams who avoid the “hope” signings have a much higher chance of success.
Even some NHL teams and scouts battle to overcome their internal biases, so it isn’t a surprise when fans and media do as well.
Recently by Jason Gregor:
Recent articles from Jason Gregor